Predicting Refractory Brick Types with our HySpex VNIR-SWIR Cameras
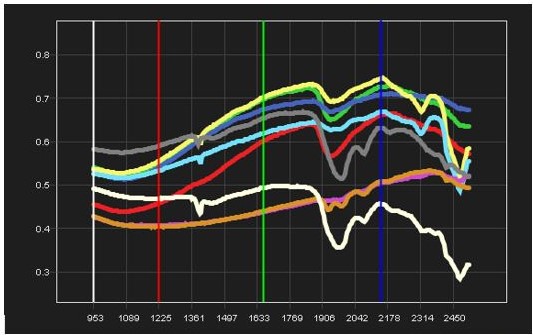
Today, we want to give you an insight in our research at NEO. Here, all of the samples we received so far were scanned in our laboratory in Oslo, Norway, using our HySpex cameras. The image below (please open in new tab) shows the general setup in the lab. We have two cameras working in different wavelength ranges, the visible to near-infrared (VNIR, 400-1000nm) and the shortwave infrared (SWIR, 1000-2500nm). They are mounted next to each other, and each collect data when the samples are being moved underneath them on the moving table. We have specific illumination sources that are active in the SWIR because normal LED lightbulbs aren’t, and we use a calibration panel for reference so we can correct the data after collection.
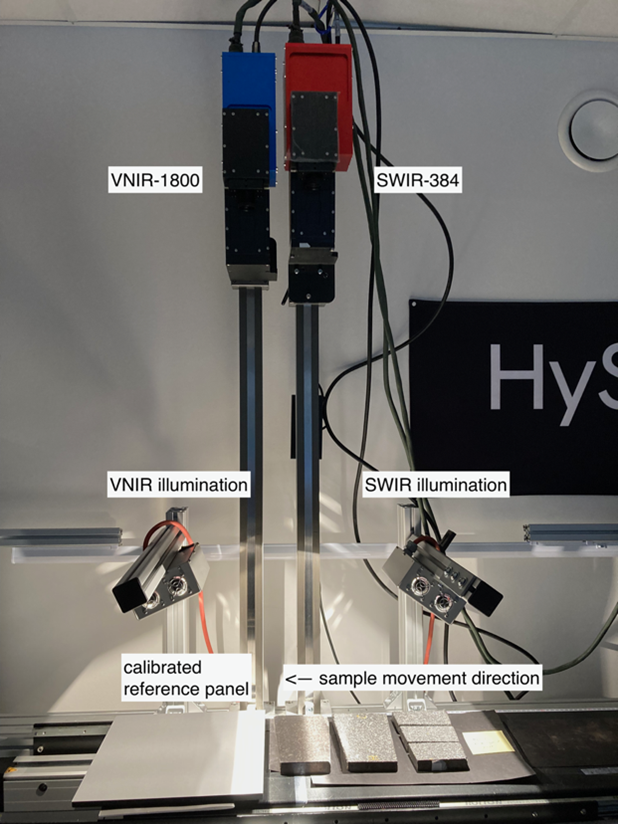
In the image below (please open in new tab), you can see that each brick represents a mixture of different spectra, depending on the pixel position within the brick. You can see that the spectral profile changes from the top (hot face) to the bottom (cold face). Material changes in the brick are caused by their closeness to the source of heat and changes in pressure. But also depending on the grain size, different areas in the brick will show different spectra. This means that the brick is not one homogeneous area but is made up of different materials that can be seen on the macro scale. Instead of trying to map the different raw materials in the brick, we buil a new model based on the brick type for each brick taking the whole brick into account and training based on the average spectra of parts of the bric We do this because on the final conveyor line in the sorting plant, we want to be able to mark different pieces of brick as a specific sorting class, instead of classifying each pixel and material indiviually. When we look at the model prediction compared to the known brick type, the model predicts the bricks correctly for 73% (SWIR) and 70% (VNIR) of the training samples. As we have a limited training sample set here (only a couple of bricks), it is likely that our models will get better over time with more samples.
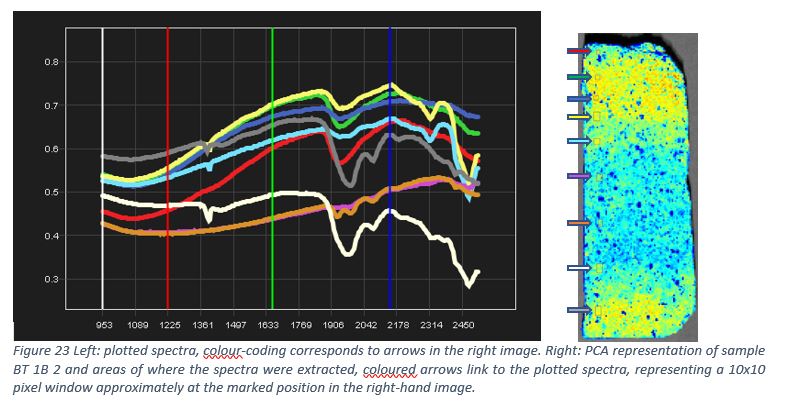
We can see that the original bricks can be classified quite well when looking at the general brick type. The example below is of a used brick from a steel casting ladle. It was identified as “fired MgO unknown” based on geochemistry and mineralogy performed by RHI Magnesita. What you can see in the image (please open in new tab) is the outside of the brick with a very dark appearance, and the inside slabs show a rather bright, white material with darker grains within. The classification of the raw materials here is quite diverse, showing aluminum (red) and different kinds of MgO components (orange, blue and yellow). The raw material classification below is based on the VNIR data, just as it was presented in the blog post about the hyperspectral imagery of the input raw materials (link). This shows the deiversity of the brick but does not allow assigning a clear brick type.
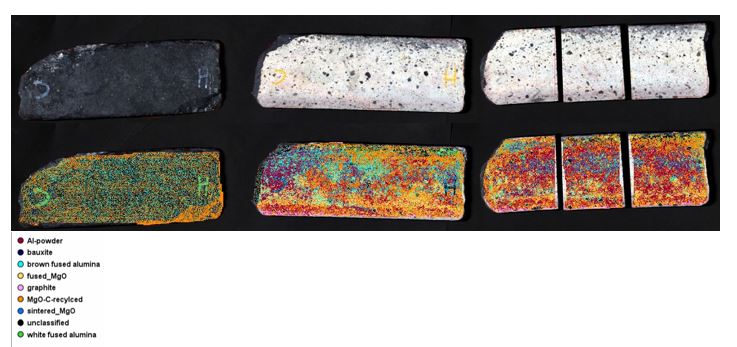
When we look at the brick composition classification, where we’re concentrating on mapping the brick type instead of the input raw materials, the pixel-based classification gives us a much clearer image. Here, we’re looking at both the VNIR and SWIR data. Please note, that the VNIR data has a higher spatial resolution, meaning that it has 4-times more pixels than the SWIR showing more small-scale changes. The input data for the modelling here is based on both the original and the used bricks provided by RHIM.
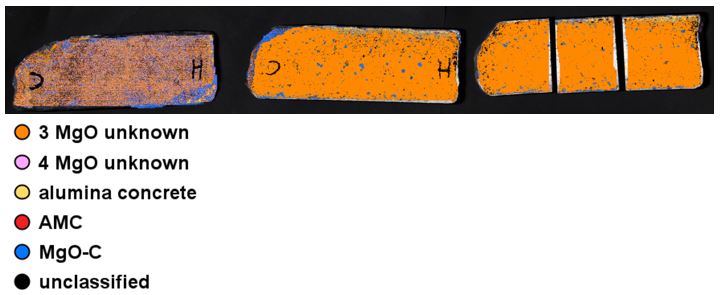
The VNIR data for this particular brick is classified dominantly as MgO unknown, reflecting the actual brick type. It’s visible that the classification has more trouble classifying the outside of the brick (left sample) as well as the edges of the brick slabs that show changes in composition because of contact changes due to e.g., heat or mortar etc. Still, the overall brick is classified as an MgO-based brick, marking it correctly.
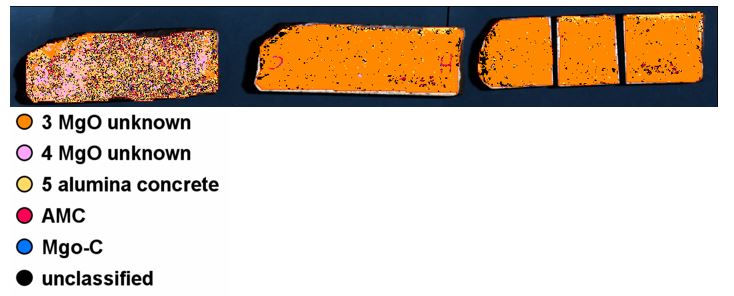
For the SWIR, the two inside slabs (middle and right) are classified quite clearly as the correct class (MgO-based brick) whereas the darker outside slab (left) is classified as a mix between MgO and alumina concrete.
These early results are promising, as they show that the different general brick types can be distinguished and classified and can aid people on site to make the correct sorting decisions.
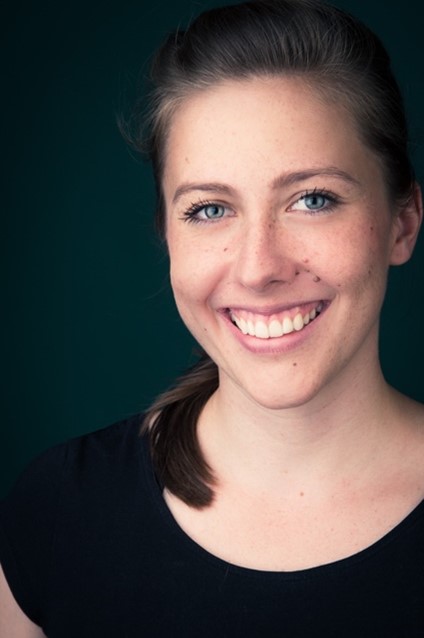
Author’s Portrait
Friederike Koerting
Dr. Friederike Koerting is working for HySpex by Norsk Elektro Optikk AS as the Geological Hyperspectral Application Specialist. HySpex by NEO is the industry-leading brand for airborne and ground-based hyperspectral imaging and is a manufacturer of advanced electro-optical products.
Friederike is applying hyperspectral imaging to help miners mitigate risks, unlock value and save time. She enjoys communicating with and to users from research and industry alike, furthering her understanding of their needs, and the possible impact of applying HySpex solutions has on users. Friederike is passionate about creating accessible platforms with a user-first mindset and establishing long-lasting channels of communication for discussions and insights. She aims to apply her expertise in the field of technology-driven sustainable mining with a regard to environmental preservation and protection. That ultimately led her to start working for HySpex in April 2021 and for her to join the ReSoURCE project activities.